Fc Barcelona Training Drills Pdf Editor
ATTACKING TACTICAL SITUATION 10 Receiving in Behind the Midfield Line and Approaching the Third Stage of Attack. ©SoccerTutor.com 94 FC Barcelona Training Sessions SESSION FOR THIS TACTICAL SITUATION (6 PRACTICES) 1. Dityach psn skachati bezkoshtovno mnusovki plyusovki pro mamu. Receive, Turn and Pass in Limited Space Objective. Jun 20, 2017 - Leicester City Football Club Academy sessions Free for coaches to take. Download link to download the entire PDF packed with sessions.
Abstract Injuries have a great impact on professional soccer, due to their large influence on team performance and the considerable costs of rehabilitation for players. Existing studies in the literature provide just a preliminary understanding of which factors mostly affect injury risk, while an evaluation of the potential of statistical models in forecasting injuries is still missing.
In this paper, we propose a multi-dimensional approach to injury forecasting in professional soccer that is based on GPS measurements and machine learning. By using GPS tracking technology, we collect data describing the training workload of players in a professional soccer club during a season. We then construct an injury forecaster and show that it is both accurate and interpretable by providing a set of case studies of interest to soccer practitioners. Our approach opens a novel perspective on injury prevention, providing a set of simple and practical rules for evaluating and interpreting the complex relations between injury risk and training performance in professional soccer. Introduction Injuries of professional athletes have a great impact on the sports industry, due to their influence on the mental state of the individuals and the performance of a team [, ]. Furthermore, the cost associated with a player’s recovery and rehabilitation is often considerable, both in terms of medical care and missed earnings deriving from the popularity of the player himself []. Recent research demonstrates that injuries in Spain cause about 16% of season absence by professional soccer players, corresponding to a cost of around 188 million euros per season [].
It is not surprising, hence, that injury forecasting is attracting a growing interest from researchers, managers, and coaches, who are interested in intervening with appropriate actions to reduce the likelihood of injuries of their players. Historically, academic work on injury forecasting has been deterred by the limited availability of data describing the physical activity of players. Nowadays, the Internet of Things have the potential to change rapidly this scenario thanks to Electronic Performance and Tracking Systems (EPTS), new tracking technologies that provide high-fidelity data streams extracted from every training and game session [, ]. These data depict in detail the movements of players on the playing field [, ] and have been used for many purposes, from identifying training patterns [] to automatic tactical analysis [,, ].
Despite this wealth of data, little effort has been put on investigating injury forecasting in professional soccer so far [,, ]. State-of-the-art approaches provide just a preliminary understanding of which variables affect the injury risk, while an evaluation of the potential of statistical models to forecast injuries is still poor. A major limit of existing studies is that they are mono-dimensional, i.e., they use just one variable at a time to estimate injury risk, without fully exploiting the complex patterns underlying the available data. Professional soccer clubs are interested in practical, usable and interpretable models as a decision making support for coaches and athletic trainers [].
In this perspective the creation of injury forecasting models poses many challenges. On one hand, injury forecasters must be highly accurate, as models which frequently produce “false alarms” are useless.
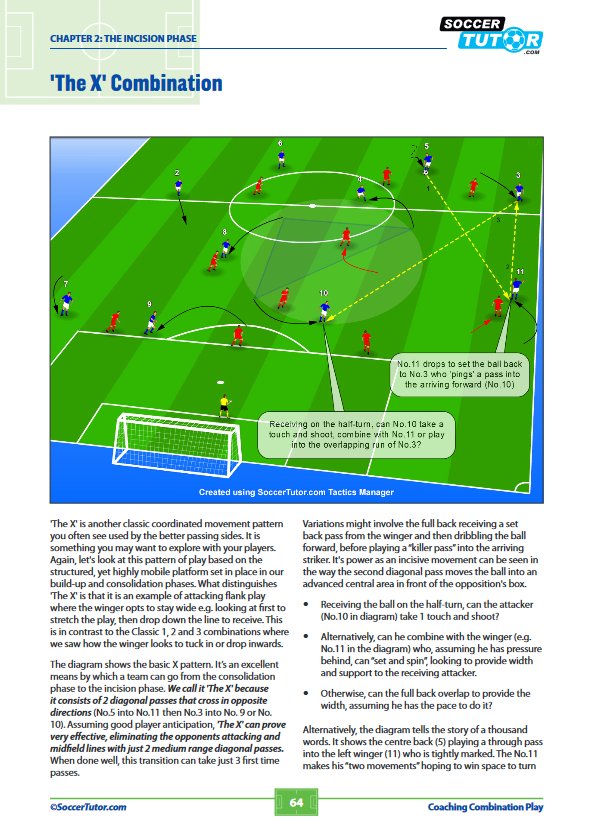
On the other hand, a “black box” approach (e.g., a deep neural network) is not desirable for practical use since it does not provide any insights about the reason behind the injuries. It goes hence without saying that injury forecasting models must achieve a good tradeoff between accuracy and interpretability. In this paper, we consider injury prediction as the problem of forecasting that a player will get injured in the next training session or official game, given his recent training workload. We observe that existing mono-dimensional approaches are not effective in practice due to their low precision (. Related work The relationship between training workload and injury risk has been widely studied in the sports science literature [,,,, ].